Artificial Intelligence and Women's Health: Innovations, Challenges, and Ethical Considerations
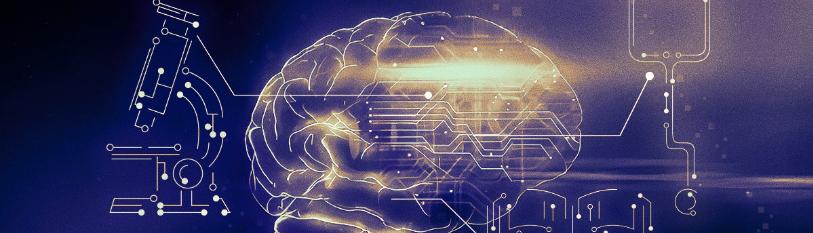
Elena Korytnikova*
Department of Obstetrics and Gynecology, Soroka University Medical Center, Faculty of Health Sciences, Ben-Gurion University of the Negev, Beer Sheva, Israel
*Corresponding author: Elena Korytnikova, Department of Obstetrics and Gynecology, Soroka University Medical Center, Faculty of Health Sciences, Ben-Gurion University of the Negev, Beer Sheva, Israel
Citation: Korytnikova E. (2023) Artificial Intelligence and Women's Health: Innovations, Challenges, and Ethical Considerations. Adv Clin Med Res. 4(3):1-6.
Received: August 8, 2023 | Published: August 29, 2023
Copyright© 2023 genesis pub by Korytnikova E. CC BY-NC-ND 4.0 DEED. This is an open-access article distributed under the terms of the Creative Commons Attribution-Non-Commercial-No Derivatives 4.0 International License., This allows others distribute, remix, tweak, and build upon the work, even commercially, as long as they credit the authors for the original creation.
DOI: https://doi.org/10.52793/ACMR.2023.4(3)-59
Abstract
Artificial Intelligence (AI) has emerged as a transformative force in various fields of healthcare, including women's health. AI applications in women's health have the potential to revolutionize disease prevention, detection and treatment. This paper explores the innovations brought by AI in women's health, discusses the difficulties associated with its implementation, and considers the ethical implications that need to be addressed.
Introduction
Women's health represents a critical aspect of public health, encompassing various conditions unique to the female anatomy and physiology. Historically, women's health research and innovation have been limited, leading to gender disparities in healthcare outcomes. However, the rise of AI applications presents opportunities to address these disparities and improve women's health outcomes. This paper discusses the potential and challenges of AI in various aspects of women's health, including reproductive health, breast cancer detection, maternal care, mental health, and personalized medicine.
AI Innovations in Women's Health
Reproductive health
AI-based applications have shown promise in revolutionizing reproductive health services. From menstrual cycle tracking and fertility prediction to contraceptive decision-making, AI instruments can provide personalized insights and recommendations based on individual health data. Additionally, AI-powered fertility treatments and assisted reproductive technologies are advancing, enhancing the chances of successful conception. A recent study reports that most participants experienced improved menstrual cycle and pregnancy knowledge through the use of the popular Flo app. The study investigated the impact of using the Flo menstrual cycle and pregnancy tracking app on women's knowledge and health by collecting survey responses from 2,212 Flo app users who had used the app for at least 30 days. Most participants reported improved knowledge about their menstrual cycle and pregnancy through app use. Users with higher education and those from high-income countries focused on pregnancy-related features, while those with lower education and from low- and middle-income countries used the app for sexual health and body awareness. The app's key components for improving knowledge were period tracking, fertile days, and ovulation predictions, suggesting menstrual health apps like Flo can contribute to global health education and empowerment [1].
Breast cancer detection
Breast cancer is a major health concern for women worldwide. AI-driven breast cancer detection tools, using image analysis and deep learning algorithms, can aid in more accurate and early diagnosis of breast cancer. Such innovations can significantly improve survival rates and reduce unnecessary biopsies. A study published in Lancet Oncology reports that implementation of artificial intelligence (AI) to analyze mammograms alongside radiologists led to the detection of 20% more cancers- and decreased doctors' mammogram reading workload almost in half [2]. This randomized, controlled trial aimed to assess the clinical safety of a AI-supported mammography screening protocol compared to standard reading by radiologists. Over 80,000 women were randomly assigned to either AI-supported screening or standard double reading. The study found that AI-supported screening had a similar cancer detection rate compared to standard reading, but with a significantly lower screen-reading workload. The trial concluded that AI in mammography screening is safe, and further assessment of the primary endpoint, interval cancer rate, is ongoing.
Another study published in 2018 in the Radiology journal aimed to compare the breast cancer detection performance of radiologists reading mammographic examinations with and without the support of an artificial intelligence (AI) system. The retrospective study involved 240 mammographic examinations interpreted by 14 radiologists. The results indicated that radiologists' diagnostic performance improved when using the AI system, with a higher area under the receiver operating characteristic curve (AUC) and increased sensitivity. The specificity also showed a trend towards improvement, and there was no significant difference in reading time. The AI system's AUC was comparable to the average AUC of the radiologists. Overall, the study suggested that AI support can enhance breast cancer detection without requiring additional reading time [3].
Maternal care
AI-enabled prenatal monitoring and risk prediction tools offer significant benefits in maternal care. Remote monitoring devices equipped with AI can recognize potential complications during pregnancy and provide timely interventions, reducing maternal mortality and improving health outcomes. A 2022 study on preeclampsia prevention published in the American Journal of Obstetrics and Gynecology aimed to develop and test an automated machine-learning model for predicting adverse outcomes in pregnant patients with suspected preeclampsia. The researchers used a real-world dataset of 1647 women and incorporated biomarker data and clinical information. They trained two different models, a gradient-boosted tree and a random forest classifier, and evaluated their performance using various metrics. The gradient-boosted tree model achieved positive predictive value of 88%, negative predictive value of 89%, sensitivity of 66%, specificity of 97%, and an overall accuracy of 89%. The study concluded that machine-learning techniques can enhance prediction of adverse outcomes in pregnant women at risk of preeclampsia compared to current clinical standards [4].
Another recent study published in the American Journal of Obstetrics and Gynecology aimed to develop a prediction model for preeclampsia using statistical learning methods based on clinical and laboratory data obtained during routine prenatal visits in early pregnancy. The retrospective cohort study included data from 16,370 births. Two algorithms, elastic net and gradient boosting, were used to build predictive models for preeclampsia and early-onset preeclampsia (<34 weeks gestation). The prediction model for preeclampsia achieved an area under the curve of 0.79, sensitivity of 45.2%, and false-positive rate of 8.1%. The model for early-onset preeclampsia achieved an area under the curve of 0.89, true-positive rate of 72.3%, and false-positive rate of 8.8%. The study concluded that these statistical learning methods effectively identified significant features and demonstrated high prediction performance for preeclampsia risk using routine early pregnancy data [5].
By analyzing complex data from electronic health records, medical images, and wearable devices, AI can recognize patterns and risk factors for pregnancy complications including preeclampsia, gestational diabetes, and preterm labor, and can help healthcare professionals intervene in a timely manner.
Mental health
Mental health is a crucial aspect of overall well-being, and women often face unique challenges related to mental health. AI-powered mental health platforms, through natural language processing and sentiment analysis, can provide personalized support, identify potential mental health issues, and offer appropriate interventions. As an example, AI has demonstrated its utility in diagnosing various types of mental disorders, often through methods inaccessible to human therapists. A recent 2020 study aimed to enhance the accuracy of short-term depression detection using mobile devices. The researchers identified five factors influencing depression from the DSM-5 (physical activity, mood, social activity, sleep, and food intake) and extracted related features using mobile device sensors. They recruited participants from different depression groups and developed a machine learning model called Short-Term Depression Detector (STDD) that utilized smartphone and wearable data for depression classification. The pilot study demonstrated strong correlations between self-reports and sensor data for various factors, achieving a 96.00% accuracy in depression group classification. This approach suggests the potential for accurate and timely depression detection using mobile devices and a promising potential of AI to transform mental health care [6].
Challenges in Implementing AI in Women's Health
Privacy and security
The use of sensitive health data in AI applications raises concerns about privacy and security. Protecting women's health data from unauthorized access, misuse, and breaches is crucial to maintain trust in AI-driven healthcare. A recent study on AI privacy and security proposed a governance model for AI applications in healthcare. The 4 main components of the suggested model are fairness, transparency, trustworthiness, and accountability [7].
Lack of regulation
The rapid evolution of AI technologies has outpaced regulatory frameworks, creating challenges in ensuring the safety and efficacy of AI applications in healthcare. Finding a balance between promoting innovation and safeguarding patients' rights is crucial.
Ethical Considerations
Informed Consent
Obtaining informed consent for using AI in women's health is essential, particularly when struggling with sensitive health issues. Women and their physicians must be fully aware of the implications of AI-driven interventions and have the right to make informed decisions about their healthcare. Astromske et al [8] suggests that the ethical audit of artificial intelligence tools including informed consent education should become an essential precondition for professional medical accreditation of future providers. The need for legal standards and legislation to address AI-related issues is also emphasized.
Transparency and explainability
AI algorithms can be very complex and hard to interpret. The lack of clarity surrounding the concept of transparency in artificial intelligence poses a significant challenge. "Black box medicine" refers to situations in healthcare where medical decisions are made by complex algorithms machine learning systems, and the reasoning behind those decisions is not readily understandable by healthcare professionals or patients.
The "Transparency Project" at the University of Cambridge addresses the challenges associated with black box medicine. The goal of this project is to develop methods and techniques that enhance the transparency and explainability of AI systems used in healthcare settings. It aims to provide meaningful explanations for the decisions made by AI algorithms, allowing healthcare professionals and patients to fully understand and trust the AI-generated recommendations [9].
Accountability and bias mitigation
Developers and healthcare providers must be accountable for the performance and outcomes of AI algorithms. Measures to detect and address bias in AI systems must be implemented to prevent discriminatory practices in women's healthcare. [7] suggests that performance and bias checks should be a shared responsibility among AI developers and healthcare providers, and should be incorporated into algorithm maintenance at regular intervals.
Equitable access
Machine learning algorithms used in healthcare have the potential to exacerbate existing health inequities. AI systems are built on historical data, which can be biased due to systemic inequalities. If AI algorithms are trained on biased data, they can intensify these biases in healthcare decision-making processes. This can lead to disparities in diagnoses, treatments, and outcomes for different demographic groups. A 2019 study published in the Science journal found that an AI-generated algorithm used to predict health care needs and guide healthcare decisions for more than 100 million people was biased against Black patients [11].
In addition, AI systems that don't account for cultural differences or language barriers can lead to misunderstandings and incorrect recommendations. This can disproportionately affect communities with different linguistic and cultural backgrounds. To harness the full potential of AI in women's health, equitable access to AI-based healthcare solutions must be ensured across different socio-economic and geographic groups.
Conclusion
AI has the potential to revolutionize women's health by providing personalized, efficient, and equitable healthcare solutions. However, to fully realize these benefits, challenges related to data bias, privacy, regulation, and ethics must be addressed. Ethical considerations and responsible use of AI technologies will play an important role in shaping a future where AI empowers and improves the lives of women in the realm of healthcare. By embracing AI innovations while honoring ethical principles, we can strive for a more inclusive and progressive healthcare system for women worldwide.
References
1. Zhaunova L, Bamford R, Radovic T, Wickham A, Peven K, et al. (2023) Characterization of Self-reported Improvements in Knowledge and Health Among Users of Flo Period Tracking App: Cross-sectional Survey. JMIR Mhealth and Uhealth. 11:e40427.
2. Lång K, Josefsson V, Larsson AM, Larsson S, Högberg C, et al. (2023) Artificial intelligence-supported screen reading versus standard double reading in the Mammography Screening with Artificial Intelligence trial (MASAI): a clinical safety analysis of a randomised, controlled, non-inferiority, single-blinded, screening accuracy study. Lancet Oncol. 24(8):936-44.
3. Roiguez-Ruiz A, Krupinski E, Mordang J, Schilling K, Heywang-Kobrunner S, et al. (2019) Detection of Breast Cancer with Mammography: Effect of an Artificial Intelligence Support System. Radiology. 290(2), 305-14.
4. Schmidt LJ, Rieger O, Neznansky M, Hackelöer M, Dröge LA, et al. (2022) A machine-learning-based algorithm improves prediction of preeclampsia-associated adverse outcomes. Am J Obstet Gynecol. 227(1):77.e1-77.
5. Marić I, Tsur A, Aghaeepour N, Montanari A, Stevenson DK, et al. (2020) Early prediction of preeclampsia via machine learning. Am J Obstet Gynecol MFM. 2(2):100100.
6. Narziev N, Goh H, Toshnazarov K, Lee SA, Chung KM, et al. (2020) STDD: Short-Term Depression Detection with Passive Sensing. Sensors (Basel). 20(5):1396.
7. Reddy Allan S, Coghlan S, Cooper P. (2020) A governance model for the application of AI in health care. J Am Med Inform Assoc. 27(3):491-97.
8. Astromskė K, Peičius E, Astromskis P. (2021). Ethical and legal challenges of informed consent applying artificial intelligence in medical diagnostic consultations. AI & Society. 36(2):509-20.
9. https://www.phgfoundation.org/report/black-box-medicine-and-transparency
10. Thomasian MN, Eickhoff C, Adashi EY. (2021). Advancing health equity with artificial intelligence. J Public Health Policy. 42(4):602-11.
11. Obermeyer Z, Powers B, Vogeli C, Mullainathan S. (2019) Dissecting racial bias in an algorithm used to manage the health of populations. Science. 366(6464):473-53.