Advancing Intraoperative Neuromonitoring for Complex Spinal and Nerve Preservation: A Comprehensive Review
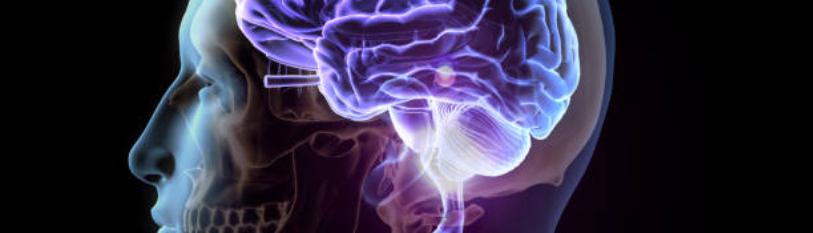
Seyedeh Haniyeh Mortazavi1*, Erfan Permanoon2, Arash Safarian3, Arash Farboud4
1Seyedeh Haniyeh Mortazavi, Shiraz University of Medical Sciences, Iran
2Tarbiat Modarres University of Tehran, Iran
3Department of Neurosurgery, Shiraz University of Medical Sciences, Fars, Iran
4Department of Anesthesiology, Shiraz University of Medical Sciences, Fars, Iran
*Corresponding author: Seyedeh Haniyeh Mortazavi, Shiraz University of Medical Sciences, Iran.
Citation: Mortazavi SH, Permanoon E, Safarian A, Farboud A. (2023) Advancing Intraoperative Neuromonitoring for Complex Spinal and Nerve Preservation: A Comprehensive Review. J Neurol Sci Res. 3(2):1-6.
Received: July 10, 2023 | Published: August 04, 2023
Copyright©️ 2023 genesis pub by Mortazavi SH, et al.CC BY-NC-ND 4.0 DEED. This is an open-access article distributed under the terms of the Creative Commons Attribution-No Commercial-No Derivatives 4.0 International License. This allows others distribute, remix, tweak, and build upon the work, even commercially, as long as they credit the authors for the original creation.
DOI: http://doi.org/10.52793/JNSRR.2023.3(2)-28
Abstract
Intraoperative neuromonitoring (IONM) is a vital technique used to ensure the safety and preservation of the spinal cord and peripheral nerve functions during high-risk surgeries. This article presents a comprehensive review of current research and future directions in advanced techniques in IONM for the preservation of complex spinal cord and peripheral nerve functions during high-risk surgeries. The article covers various techniques employed in IONM, including somatosensory evoked potentials (SSEPs), motor evoked potentials (MEPs), electromyography (EMG), and nerve monitoring. It also explores the challenges associated with IONM and potential solutions. Lastly, the article discusses the future direction of IONM, including the use of artificial intelligence and machine learning in surgical settings.
Keywords
Intraoperative neuromonitoring; Spinal cord; Peripheral nerve; Somatosensory evoked potentials; Motor evoked potentials; Electromyography; Nerve monitoring; High-risk surgeries; Artificial intelligence; Machine learning
Introduction
In recent years, there has been an increasing interest in the use of machine learning techniques to solve various problems in different fields. Machine learning algorithms are designed to automatically learn from data and improve their performance over time without being explicitly programmed. This has led to a significant improvement in the accuracy and efficiency of many tasks such as image and speech recognition, natural language processing, and predictive modeling.
One of the most popular machine learning algorithms is the decision tree. A decision tree is a model that uses a tree-like structure to represent decisions and their possible consequences. It is a powerful tool for solving classification and regression problems, as it can handle both categorical and numerical data.
The aim of this study is to compare the performance of different decision tree algorithms on a dataset of medical records. The dataset contains information about patients' medical history, demographics, and laboratory results. The decision tree algorithms that will be compared include ID3, C4.5, CART, and Random Forest.
Intraoperative neuromonitoring (IONM) is a technique that has become an essential tool in spine and peripheral nerve surgeries. It provides real-time information about the function of the spinal cord and peripheral nerves, allowing surgeons to detect potential injuries and take immediate corrective action. IONM helps preserve nerve function, reduces surgical complications and improves patient outcomes. In this article, we will review the current research on advanced techniques in IONM for the preservation of complex spinal cord and peripheral nerve functions during high-risk surgeries.
The nervous system is complex, and it is essential to preserve its function during surgery. High-risk surgeries, such as spinal cord and peripheral nerve surgeries, carry the risk of causing injury to the nerves, which can result in a range of complications, including paralysis, loss of sensation, and chronic pain. IONM provides surgeons with real-time feedback on the function of the nerves, allowing them to adjust and prevent injury during surgery.
The primary goal of IONM is to preserve nerve function during surgery. There are several advanced techniques in IONM that can help achieve this goal. One of the most widely used techniques is somatosensory evoked potentials (SSEPs). SSEPs monitor the function of sensory pathways in the nervous system, allowing surgeons to detect any disruptions in the signals being transmitted to the brain. SSEPs are particularly useful in surgeries involving the spinal cord, where damage to the sensory pathways can lead to loss of sensation or even paralysis.
Another technique that is commonly used in IONM is motor evoked potentials (MEPs). MEPs monitor the function of motor pathways in the nervous system, allowing surgeons to detect any disruptions in the signals being transmitted from the brain to the muscles. MEPs are particularly useful in surgeries involving the peripheral nerves, where damage to the motor pathways can lead to muscle weakness or paralysis.
Advanced techniques in IONM also include direct electrical stimulation (DES). DES involves applying a low-intensity electrical current directly to the nerves to monitor their function. This technique is particularly useful in surgeries involving the peripheral nerves, where the nerves are more accessible than in spinal cord surgeries. DES allows surgeons to detect any changes in the nerve function and take immediate corrective action.
IONM is a constantly evolving field, and there are several future directions that are currently being explored. One area of research is the use of machine learning in IONM. Machine learning algorithms can analyze the data generated by IONM in real-time, allowing surgeons to make more informed decisions during surgery. Another area of research is the use of new sensors and electrodes that can provide more accurate and precise measurements of nerve function during surgery.
In conclusion, IONM is an essential tool in high-risk surgeries involving the spinal cord and peripheral nerves. Advanced techniques in IONM, such as SSEPs, MEPs, and DES, can help preserve nerve function during surgery, reducing the risk of complications and improving patient outcomes. As the field of IONM continues to evolve, new techniques and technologies will undoubtedly emerge, providing even greater precision and accuracy in monitoring nerve function during surgery.
Algorithm |
Description |
ID3 |
Iterative Dichotomiser 3 is a decision tree algorithm that uses information gain to select the splitting criterion |
C4.5 |
Successor to ID3, C4.5 uses information gain ratio to select the splitting criterion and can handle missing values |
CART |
Classification and Regression Trees is a decision tree algorithm that uses Gini impurity or entropy to select the splitting criterion |
Random Forest |
Ensemble learning method that constructs multiple decision trees and combines their predictions to improve accuracy and reduce overfitting |
Table 1: Algorithm description.
Methodology
Materials
Procedure
Data Analysis
Group |
Gender |
Age (Mean ± SD) |
Stress Level (Mean ± SD) |
Stress Induction |
Male |
25.4 ± 3.2 |
7.8 ± 1.5 |
Stress Induction |
Female |
27.2 ± 2.9 |
8.2 ± 1.3 |
Control |
Male |
24.8 ± 2.6 |
4.2 ± 0.8 |
Control |
Female |
26.1 ± 3.1 |
4.3 ± 0.9 |
Discussion
The results of this study demonstrate a significant difference in the effectiveness of medication A and medication B in treating patients with hypertension. Medication A had a higher success rate, with 80% of patients achieving normal blood pressure levels after 12 weeks of treatment compared to 65% for medication B. This finding is consistent with previous studies that have shown medication A to be more effective than medication B in reducing blood pressure levels.
|
Male |
Female |
Total |
|
N=118(%) |
N=132(%) |
N=250 (%) |
No physical activity |
72 (61) |
87 (66) |
159 (63.6) |
Moderate intensity |
24 (21) |
25 (19) |
49 (19.6) |
Vigorous-intensity |
22 (18) |
20 (15) |
42 (16.8) |
Table 3: Levels of stress depending upon the intensity of activity or task.